INTRODUCTION
Chronic ankle instability (CAI) is a multifaceted condition that frequently emerges as a consequence of a lateral ankle sprain. This disorder is defined by ongoing symptoms that persist for over a year after the initial injury, including discomfort, inflammation, reduced self-reported functionality, and recurring episodes or sensations of ankle instability, often accompanied by repeated ankle sprains.1-3 The occupational demands placed on logistics workers (LWs) make them particularly susceptible to ankle sprains. Their job typically requires traversing an average of 8 kilometers per shift while handling packages of diverse dimensions, weights, and configurations.4 In the spectrum of work-related musculoskeletal disorders, ankle sprains rank as the second most prevalent issue.5 Moreover, among LWs, the ankle is the most injured body part, accounting for 23% of all injuries.6 The risk of ankle injuries in this population is exacerbated by the unpredictable and varied outdoor environments in which deliveries are made, often under uncontrolled conditions.7
CAI is a common condition characterized by recurrent ankle sprains and persistent symptoms following an initial ankle sprain.8 Individuals with CAI often exhibit impaired postural control and dynamic balance, which are critical components of functional stability and injury prevention.9-12 The relationship between CAI and deficits in postural control and dynamic balance has been well-documented in the literature, with studies showing that individuals with CAI demonstrate decreased performance in various balance tasks compared to healthy controls.13,14 These impairments can significantly impact daily activities and increase the risk of future injuries, particularly in occupations that require prolonged standing or frequent movement, such as LWs.
The Y-Balance Test (YBT) has emerged as a valuable tool for assessing dynamic balance and identifying individuals at risk for lower extremity injuries, including those with CAI.15,16 The YBT is a modification of the Star Excursion Balance Test and requires participants to maintain single-leg stance while reaching as far as possible with the contralateral leg in three directions: anterior, posteromedial, and posterolateral.17 The test provides quantitative data on reach distances and allows for the calculation of a composite score, offering clinicians a standardized method to evaluate dynamic balance and functional symmetry. Several studies have investigated the use of cut-off values for the YBT to identify individuals with CAI or those at risk of lower extremity injuries. Plisky et al. (2006) proposed that high school basketball players with an anterior reach asymmetry greater than 4 cm were at 2.5 times greater risk of lower extremity injury.15 Butler et al. (2013) found that collegiate football players with a composite YBT score less than 89.6% of limb length were at increased risk for non-contact lower extremity injuries.18 However, the applicability of these cut-off values to different populations, such as LWs, remains uncertain, and there is a need for population-specific cut-off values to improve the clinical utility of the YBT.
In recent years, machine learning techniques have been increasingly applied in sports and rehabilitation sciences to identify patterns and classify data. K-means clustering, an unsupervised learning algorithm, has been used in sports and ergonomics related studies based on performance characteristics, musculoskeletal pain or injury risk factors.19-21 While traditional methods like Youden’s J statistic have been widely used to determine cut-off values, the application of K-means clustering for this purpose offers a novel approach.22-24 By identifying the optimal number of clusters and using the midpoint between the top two clusters as the cut-off value, K-means clustering may provide a more data-driven and nuanced approach to establishing YBT cut-off values.
Ankle sprains result in substantial workplace disruption, with an average of 20 lost workdays per incident.5 The situation is further compounded by recurrent sprains associated with CAI, which can lead to even greater productivity losses. Given these significant impacts, there is a pressing need for accurate classification of LWs with and without CAI.25 This classification is crucial not only for effective condition management but also for facilitating timely return to work. However, the demanding nature of the logistics service environment necessitates the use of simple, quick, and reliable assessment tools that can be easily administered in the field. Therefore, research exploring the application of easily implementable tools like the YBT for accurate CAI classification in workplace settings is essential. Such research could provide valuable insights for occupational health professionals and employers in their efforts to mitigate the impact of ankle injuries and CAI on LWs and overall workplace productivity. The purpose of this study is to compare two methods for determining YBT cut-off values in LWs with a history of ankle sprains: (1) a novel approach using K-means clustering, and (2) the traditional Youden’s J statistic method. By exploring the potential of K-means clustering in determining YBT cut-off values, we aim to enhance the accuracy of CAI identification and improve the clinical utility of the YBT for LWs with a history of ankle sprains. This research contributes to the growing body of literature on the application of machine learning techniques in clinical assessment and may provide valuable insights into the development of population-specific cut-off values for dynamic balance tests.
METHODS
The study utilized data from musculoskeletal screening tests conducted at a healthcare center for a logistics company between August 2021 and March 2022. These tests were originally performed to prevent industrial accidents among LWs. Due to the retrospective nature of the analysis using pre-existing company data, the Institutional Review Board waived the requirement for informed consent (approval number: 1041849-202301-BM-016-01). From a pool of 289 LWs, 121 individuals with a history of at least one ankle sprain were identified as potential participants. CAI was defined according to specific criteria: a history of lateral ankle sprain causing pain and impaired physical function, followed by at least one episode of perceived instability or ‘giving way’.26 The ankle instability instrument was employed to confirm recurrent instability, with a minimum score of 5 points required for inclusion.26 The study excluded LWs with less than 6 months of work experience in logistics service. Additionally, individuals were excluded if they had undergone lower-extremity surgery within the past 6 months, had been diagnosed with ankle osteoarthritis, or had a history of ankle surgery involving intra-articular fixation.
The examiner used the Y-Balance Test Kit.16,17 The device consists of a single central plastic plate and three attached tubes arranged in anterior, posteromedial, and posterolateral positions. A measure is positioned on each of the tubes, with an interval of 0.5 cm. The subjects, while standing on the affected leg (barefoot) in a central location on the YBT instrument, with hands placed on the wing of the ilium, were asked to move the pointer as far as possible, using the lower limb opposite to the support limb, in three directions e anterior, posteromedial, posterolateral. All YBT attempts were performed in the same order: the first e anterior direction; the second - posterolateral direction; and the last e posteromedial direction. Participants performed six familiarization trials followed by two recorded trials, maintaining an upright posture with hands on their chest throughout the reaches. The YBT composite score was derived by summing the maximum reach distances in each direction and dividing by three times the limb length.
The data analysis and statistical procedures were conducted using Python (version 3.8.5) with scikit-learn (version 0.24.2), scipy (version 1.6.2), and Orange (version 3.28.0) libraries. Our analysis focused on four key variables from the YBT: anterior direction distance, posterolateral direction distance, posteromedial direction distance, and the composite score. This comprehensive methodology provides a robust framework for determining and evaluating cut-off values (Youden’s J Statistic and K-means Clustering) in the four key YBT measures for CAI classification, with a specific focus on establishing stringent criteria for industrial accident prevention and safe return to work among LWs with a history of ankle sprains with a history of ankle sprains. By combining a traditional statistical approach with an advanced machine learning technique tailored to our specific needs, we aim to offer new insights into the classification of CAI using YBT measures, ultimately contributing to improved workplace safety protocols.
Prior to analysis, the dataset underwent rigorous preprocessing. Missing values in the four YBT variables were addressed using mean imputation via scikit-learn’s SimpleImputer class. This method replaces missing values with the mean value of the respective variable, helping to maintain the overall distribution of the data. Subsequently, these variables were standardized using z-score normalization (μ=0, σ=1) using StandardScaler. The distribution of each variable was confirmed as a boxplot to remove outliers using a local outlier factor (contamination=10%; neighbors =20; metric=Euclidean) because it influences the accuracy of the learning model.
To determine cut-off values for each of the four YBT variables, we employed two distinct methods: Youden’s J Statistic and K-means Clustering. The Youden’s J Statistic is a well-established method in clinical research that balances sensitivity and specificity. For each YBT variable independently, we determined the optimal cut-off point by maximizing the Youden’s J statistic (J=Sensitivity+Specificity–1). This involved computing the receiver operating characteristic (ROC) curve for each YBT variable, calculating the Youden’s J statistic for each point on the ROC curve, and identifying the threshold that maximizes the J statistic for each variable. We also computed the area under the ROC curve (AUC) to assess the discriminative ability of each YBT measure. In addition to this traditional approach, we introduced a novel method based on K-means clustering, specifically designed to establish more stringent cut-off values. K-means clustering is an unsupervised machine learning technique that aims to partition n observations into k clusters, where each observation belongs to the cluster with the nearest mean (centroid). In our context, this allows us to identify natural groupings in the YBT data that may correspond to different levels of ankle stability.
For the K-means Clustering method, we first determined the optimal number of clusters (k) using the silhouette score. The silhouette score measures how similar an object is to its cluster compared to other clusters, with scores ranging from –1 to 1. A higher silhouette score indicates better-defined clusters. We computed this score for k ranging from 2 to 10 to find the optimal number of clusters for our data. Once the optimal k was determined, we applied K-means clustering to the standardized data of all four YBT variables collectively. This approach allows us to consider the interrelationships among the YBT measures, potentially capturing more complex patterns than analyzing each variable independently. After clustering, we computed the centroids (mean values) of each cluster and inverse-transformed them to the original scale. Once the optimal k was determined, we applied K-means clustering to the standardized data of all four YBT variables collectively. This approach allows us to consider the interrelationships among the YBT measures, potentially capturing more complex patterns than analyzing each variable independently. After clustering, we computed the centroids (mean values) of each cluster and inverse-transformed them to the original scale. For each YBT variable, the cut-off value was calculated as the mean of all cluster centroids for that specific variable.
The K-means Clustering (Top 2) method builds upon the previous approach but focuses on the upper range of the data distribution. Using the same optimal k value and cluster assignments from the K-means (Mean) method, we sorted the cluster centroids in ascending order for each YBT variable independently. The cut-off value for each variable was then calculated as the mean of the top two cluster centroids for that specific variable. This method aims to identify a threshold that separates the higher-performing individuals, which may be more relevant for distinguishing those with CAI.
For each method and each of the four YBT variables, we calculated a comprehensive set of performance metrics. Sensitivity and specificity were computed based on the confusion matrix derived from the predicted and actual CAI status for each YBT variable and method. We employed Fisher’s exact test to calculate the odds ratio for each YBT variable and method, providing a measure of association between the dichotomized YBT scores and CAI status. The 95% confidence interval (CI) of the odds ratio was estimated using the standard error of the log odds ratio, which provides a more accurate CI for small sample sizes compared to the normal approximation method. The area under the curve (AUC) was calculated for both methods. For Youden’s J statistic method, the AUC was directly computed from the ROC curve for each YBT variable. For the K-means method, which produces binary predictions based on the derived cut-off values, we calculated the AUC using these predictions. To facilitate comparison, we systematically evaluated the performance of each method (Youden’s J statistic and K-means) across all four YBT variables. A comprehensive results table was generated, including cut-off values, AUC, sensitivity, specificity, odds ratio, and the 95% CI of the odds ratio for each method and YBT variable. This tabular format allows for direct comparison and identification of the most effective cut-off determination method for each YBT measure, with particular attention to the stricter criteria established by the K-means method.
RESULTS
Table 1 shows the average values and variability of all the variables. The Kolmogorov-Smirnov normality test presented normally distributed data of all the independent variables (p>0.05). Our study evaluated 121 DSW who had a history of ankle sprains with a history of ankle sprains with and without CAI and were analyzed using ML models. There were no significant differences between LWs who had a history of ankle sprains with and without CAI in terms of age, work duration, YBT composite score, and YBT anterior direction distance. The BMI, Ankle Instability Instrument score, and RCSP in LWs who had a history of ankle sprains with CAI were statistically higher than that in LWs who had a history of ankle sprains without CAI. The YBT posteromedial and posterolateral direction distances in LWs who had a history of ankle sprains with CAI were statistically lower than those in LWs who had a history of ankle sprains without CAI. All the LWs who had a history of ankle sprains in the present study were male. The proportions of LWs who had a history of ankle sprains with CAI on the right, left or both sides were 28.6% (n=16), 26.8% (n=15), or 44.6% (n=25; the side with the greater Ankle Instability Instrument score: right=8 and left=17), respectively. The proportions of LWs who had a history of ankle sprains without CAI having their involved side as the right, left or both sides were 47.7% (n=31), 29.2% (n=19), or 23.1% (n=15; the side with the greater history of ankle lateral sprain: right=5 and left=10), respectively.
The silhouette score was the greatest when the number of clusters was 3 (silhouette score=0.534) from 2 to 10. Consequently, the optimal number of clusters for k-means clustering was 3. Table 2 presents the three YBT variable clusters identified using the k-means algorithm clustering based on YBT anterior direction distance, posterolateral direction distance, posteromedial direction distance, and the composite score. Among 112 PDWs who had a history of ankle sprains, Cluster 1 (C1) comprised the majority of participants (N=68) and demonstrated intermediate YBT scores. Cluster 2 (C2, N=20) showed the lowest performance across all YBT measures, while Cluster 3 (C3, N=22) exhibited the highest scores. A significant difference in YBT composite score (F=291.143, p<0.001), YBT anterior direction distance (F=89.268, p<0.001), YBT posteromedial direction distance (F=217.666, p<0.001), and YBT posterolateral direction distance (F=162.864, p<0.001) was observed among the three clusters.
The study compared three methods for determining cut-off values in YBT measures to classify CAI in LWs with a history of ankle sprains: Youden’s J statistic, K-means clustering using the mean of all centroids (K-means Mean), and K-means clustering using the mean of the top two centroids (K-means Top 2). The results for each YBT measure are as follows (Table 3 and Figure 1):
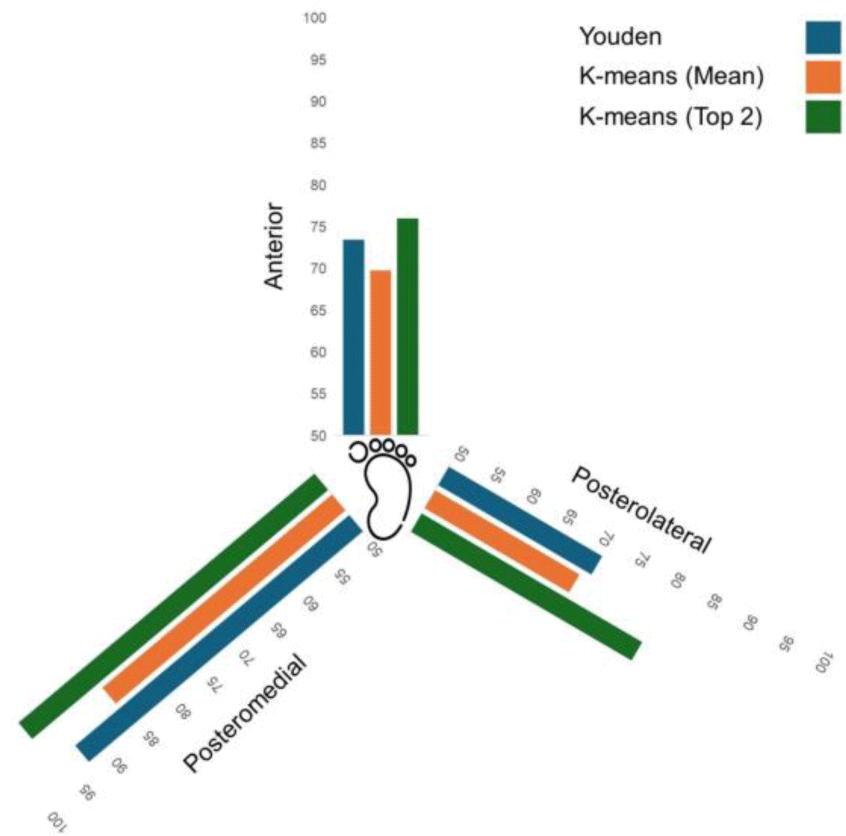
For the YBT composite score, Youden’s method yielded a cut-off value of 85.99%, with an AUC of 0.61, sensitivity of 0.66, specificity of 0.57, and an odds ratio of 2.62 (95% CI: 1.21–5.68). The K-means Mean method produced similar results with a cut-off of 86.14%, AUC of 0.61, sensitivity of 0.64, and specificity of 0.57 (OR: 2.43, 95% CI: 1.13–5.23). The K-means Top 2 method resulted in a higher cut-off (96.98%) with lower sensitivity (0.30) but higher specificity (0.87) and a comparable odds ratio (2.93, 95% CI: 1.10–7.78).
For the YBT anterior direction distance (Figure 2), Youden’s method established a cut-off at 73.37, with an AUC of 0.57, sensitivity of 0.38, and specificity of 0.80 (OR: 2.35, 95% CI: 1.00–5.52). The K-means methods showed lower performance, with the K-means Mean method yielding a lower cut-off (69.70) and the K-means Top 2 method providing a higher cut-off (75.98) but with reduced sensitivity (0.27) and increased specificity (0.83).
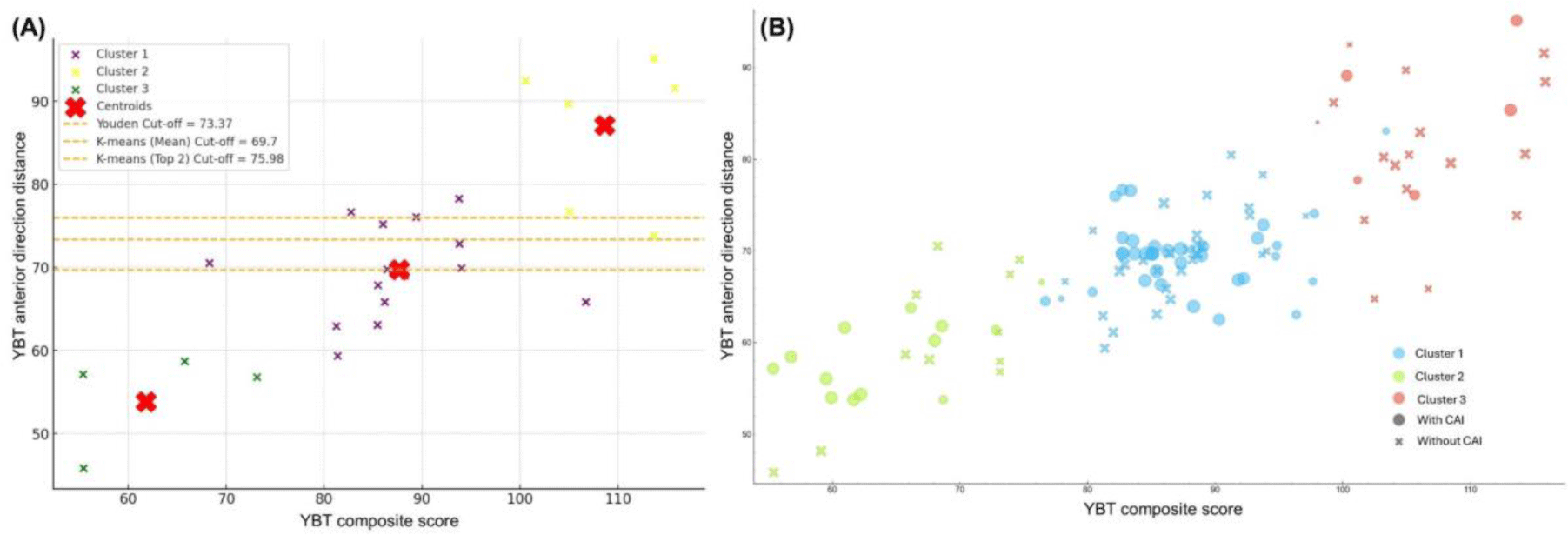
In the YBT posterolateral direction distance (Figure 3), Youden’s method determined a cut-off of 71.26, achieving an AUC of 0.59, sensitivity of 0.57, and specificity of 0.63 (OR: 2.27, 95% CI: 1.05–4.87). The K-means methods showed similar patterns to the anterior direction, with the K-means Top 2 method providing a higher cut-off (80.56) but lower sensitivity (0.34) and higher specificity (0.81).
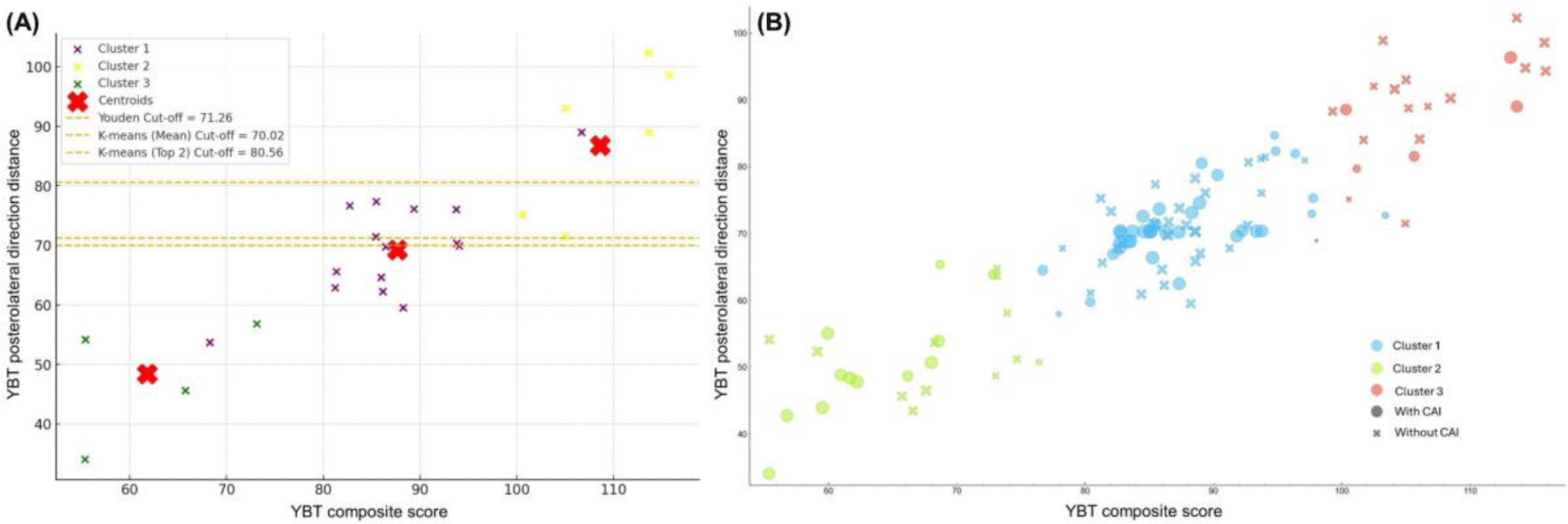
For the YBT posteromedial direction distance (Figure 4), Youden’s method established a cut-off at 92.86, demonstrating the highest AUC (0.62) and odds ratio (5.41, 95% CI: 2.09–14.04) among all measures and methods. It achieved a sensitivity of 0.45 and a high specificity of 0.87. The K-means Mean method resulted in a lower cut-off (85.93) with more balanced sensitivity (0.57) and specificity (0.59), while the K-means Top 2 method yielded a higher cut-off (96.31) with lower sensitivity (0.29) but maintained high specificity (0.87).
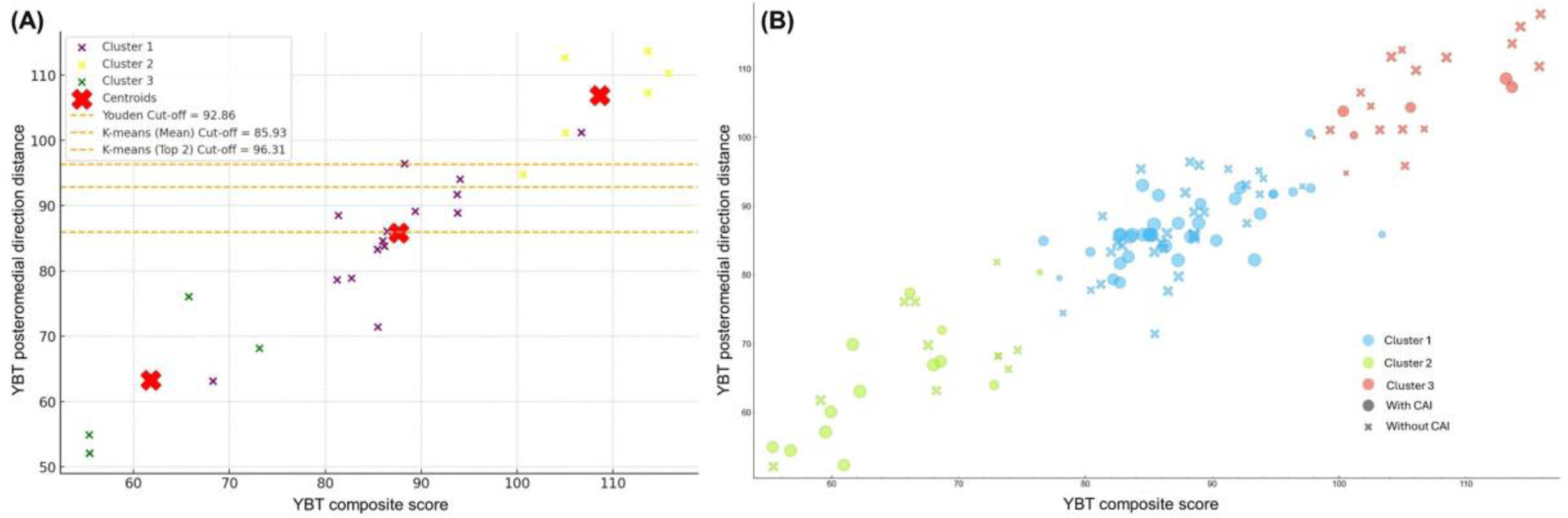
Overall, these results suggest that the YBT posteromedial direction distance, particularly when using Youden’s method for cut-off determination, may be the most effective measure for classifying CAI in LWs with a history of ankle sprains. The K-means Top 2 method consistently provided higher cut-offs with improved specificity across all YBT measures, which may be valuable for identifying individuals at higher risk of CAI in occupational settings where more stringent criteria are required based on the results of specificity.
DISCUSSION
This study aimed to compare the effectiveness of K-means clustering and Youden’s J statistic in determining YBT cut-off values for classifying CAI in LWs with a history of ankle sprains. Our findings suggest that while both methods can identify those at risk of CAI, they offer different trade-offs between sensitivity and specificity. This research contributes to the growing body of literature on the application of machine learning techniques in clinical assessment and provides valuable insights into the development of population-specific cut-off values for dynamic balance tests.
Comparing our results to previous studies, we found some notable differences in cut-off values. For instance, Butler et al.18 reported a cut-off value of 89.6% for the YBT composite score in collegiate American football players for classifying non-contact lower extremity injuries, while our study found a lower cut-off value of 85.99% using Youden’s J statistic for classifying CAI in LWs.18 This lower cut-off in our study can be attributed to the differences in the study population, as LWs likely have different physical demands and characteristics compared to collegiate athletes. Similarly, Plisky et al.15 suggested a cut-off of 94% for high school basketball players to identify those at risk of lower extremity injury.15 The variation in these cut-off values highlights the importance of developing population-specific thresholds, as the demands of different occupations and sports may significantly influence optimal cut-off points for identifying injury risk. Notably, our study goes beyond previous research by employing K-means clustering, which allowed us to consider all three directions of the YBT (anterior, posteromedial, and posterolateral) simultaneously, along with the composite score. This comprehensive approach resulted in cut-off values ranging from 85.99% to 96.98%, depending on the method used. The inclusion of all YBT directions in determining cut-offs represents a significant advancement in the field, as it provides a more holistic assessment of dynamic balance and potentially more accurate risk classification. This multi-directional approach aligns with the recommendations of Gonell et al.27, who emphasized the need for comprehensive, sport-specific YBT cut-off values in their study on soccer players for predicting soft tissue injuries.27
The discrepancy in AUC values between K-means clustering and Youden’s J statistic methods can be attributed to their fundamental differences in approach and data utilization. Youden’s J statistic is a traditional approach that balances sensitivity and specificity22,24 and calculates cut-off values for each YBT variable independently. In contrast, K-means clustering is an unsupervised machine learning technique that identifies natural groupings in the data20,28 and considers all four YBT variables (anterior, posteromedial, posterolateral, and composite scores) simultaneously to create comprehensive classifications of dynamic balance. This holistic approach allows K-means to capture more complex patterns and interactions among the YBT variables, potentially leading to more nuanced risk classifications. The K-means approach, particularly the Top 2 method, consistently provided higher cut-offs with improved specificity across all YBT measures. This difference in performance may be due to K-means’ ability to account for the interrelationships between YBT variables, potentially identifying high-risk individuals more accurately at the cost of lower sensitivity. This finding is consistent with recent studies that have explored machine learning techniques for injury prediction in sports medicine and ergonomics, which often benefit from considering multiple variables simultaneously.20,21,29-31
In an industrial setting, where the prevention of CAI is crucial for maintaining worker productivity and reducing workplace injuries, the use of higher cut-off values as provided by the K-means Top 2 method may be particularly valuable. This approach is especially relevant given the substantial workplace disruption caused by ankle sprains, with an average of 20 lost workdays per incident.5 The situation is further exacerbated by recurrent sprains associated with CAI, which can lead to even greater productivity losses. While this approach may result in more false positives, it allows for the identification of a larger proportion of at-risk individuals. This conservative approach could be beneficial in occupational health settings where the cost of missing a potential injury is high, and implementing preventive measures for a larger group is feasible and cost-effective in the long run. The YBT, as a simple, quick, and reliable assessment tool, addresses the need for easily implementable measures in the demanding logistics service environment. Its application for accurate CAI classification in workplace settings is essential, as it can be readily administered in the field. By using the K-means clustering method with the YBT, we provide a more comprehensive approach to risk assessment for thorough screening and preventive strategies.10,16 This research offers valuable insights for occupational health professionals and employers in their efforts to mitigate the impact of ankle injuries and CAI on LWs and overall workplace productivity. By facilitating early identification of at-risk workers, this approach can contribute to more effective condition management and timely return to work, ultimately reducing the economic burden associated with ankle injuries in the logistics industry.
However, this study has several limitations that should be addressed in future research. Firstly, our sample size was relatively small, which may limit the generalizability of our findings. Secondly, we only examined LWs, and the results may not be applicable to other occupations or athletic populations. Future studies should investigate larger and more diverse populations to validate these findings.32 Additionally, we did not consider other potential risk factors for CAI, such as previous injury history or biomechanical factors, which could influence the accuracy of our cut-off values. Longitudinal studies that track individuals over time would provide more robust evidence for the predictive validity of these cut-off values in sporting populations.33 Lastly, while we compared two methods for determining cut-off values, future research could explore other advanced statistical and machine learning techniques to further improve the accuracy of CAI risk classification.
CONCLUSIONS
The present study compared K-means clustering and Youden’s J statistic in determining YBT cut-off values for classifying CAI in LWs with a history of ankle sprains. Our findings revealed that the YBT posteromedial direction distance, using Youden’s method, demonstrated the highest discriminative ability for CAI classification, with an AUC of 0.62 and an odds ratio of 5.41 (95% CI: 2.09–14.04). The K-means Top 2 method consistently provided higher cut-offs with improved specificity across all YBT measures, notably achieving 87% specificity for the YBT composite score (cut-off: 96.98%). These results highlight the potential of machine learning techniques in developing more nuanced, population-specific cut-off values for dynamic balance tests, which could be particularly valuable in occupational health settings where stringent screening criteria are necessary.